Research Highlights
In predictive modeling, bigger is better
—
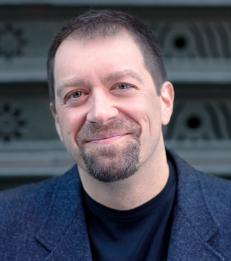

It is striking how quaint the massive data sets of yesteryear look today.
By Foster Provost
The ability to make predictions about consumer behavior is one of the benefits of big data analysis, but exactly what kind of data, how much data, and how detailed that data should be are open questions that many firms are eager to have answered. NYU Stern Professor of Information Systems and NEC Faculty Fellow Foster Provost co-wrote a book on the subject last year, called Data Science for Business: What You Need to Know About Data Mining and Data-Analytic Thinking. Now he has published new research indicating that in many instances, companies would do well to collect, curate, and model from the largest data sets they can manage.
In “Predictive Modeling with Big Data: Is Bigger Really Better?,” Provost and co-authors Enric Junque de Fortuny and David Martens explore whether more data actually leads to better predictive models. Increases in computing power and memory, they note, as well as improved algorithms and algorithmic understanding, now enable models to be built from very large data sets. Said Provost, “It is striking how quaint the massive data sets of yesteryear look today.”
The authors researched nine different predictive modeling applications, from book reviews to banking transactions, with a particular focus on the highly detailed feature data derived from the observation of individuals’ behaviors, such as prior purchases, and including such information as demographic, geographic, and psychographic characteristics. This level of information is increasingly available, Provost pointed out, as people’s online activity is logged whenever they use their smart phones, make financial transactions, surf the Web, send e-mail, tag online photos, and post ‘‘likes’’ on social media, for example.
Increasing the data set to a massive size makes predictive modeling with transactional data “substantially more accurate,” the authors concluded, “implying that institutions with larger data assets – plus the ability to take advantage of them – potentially can obtain substantial competitive advantage over institutions without access to so much data, or without the ability to handle it.”
In “Predictive Modeling with Big Data: Is Bigger Really Better?,” Provost and co-authors Enric Junque de Fortuny and David Martens explore whether more data actually leads to better predictive models. Increases in computing power and memory, they note, as well as improved algorithms and algorithmic understanding, now enable models to be built from very large data sets. Said Provost, “It is striking how quaint the massive data sets of yesteryear look today.”
The authors researched nine different predictive modeling applications, from book reviews to banking transactions, with a particular focus on the highly detailed feature data derived from the observation of individuals’ behaviors, such as prior purchases, and including such information as demographic, geographic, and psychographic characteristics. This level of information is increasingly available, Provost pointed out, as people’s online activity is logged whenever they use their smart phones, make financial transactions, surf the Web, send e-mail, tag online photos, and post ‘‘likes’’ on social media, for example.
Increasing the data set to a massive size makes predictive modeling with transactional data “substantially more accurate,” the authors concluded, “implying that institutions with larger data assets – plus the ability to take advantage of them – potentially can obtain substantial competitive advantage over institutions without access to so much data, or without the ability to handle it.”