Opinion
The Long Road to Fairer Algorithms
—
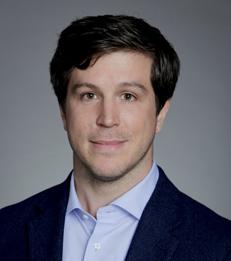

Such decentralization is expected to bring cost savings and empowerment. If it fails to materialize, we return to the problems of power and trust.
By Joshua Loftus and Matt Kusner
An algorithm deployed across the United States is now known to underestimate the health needs of black patients1. The algorithm uses health-care costs as a proxy for health needs. But black patients’ health-care costs have historically been lower because systemic racism has impeded their access to treatment — not because they are healthier.
This example illustrates how machine learning and artificial intelligence can maintain and amplify inequity. Most algorithms exploit crude correlations in data. Yet these correlations are often by-products of more salient social relationships (in the health-care example, treatment that is inaccessible is, by definition, cheaper), or chance occurrences that will not replicate.
To identify and mitigate discriminatory relationships in data, we need models that capture or account for the causal pathways that give rise to them. Here we outline what is required to build models that would allow us to explore ethical issues underlying seemingly objective analyses. Only by unearthing the true causes of discrimination can we build algorithms that correct for these.
Read the full Nature article.
___
Joshua Loftus is Assistant Professor of Information, Operations and Management Sciences
This example illustrates how machine learning and artificial intelligence can maintain and amplify inequity. Most algorithms exploit crude correlations in data. Yet these correlations are often by-products of more salient social relationships (in the health-care example, treatment that is inaccessible is, by definition, cheaper), or chance occurrences that will not replicate.
To identify and mitigate discriminatory relationships in data, we need models that capture or account for the causal pathways that give rise to them. Here we outline what is required to build models that would allow us to explore ethical issues underlying seemingly objective analyses. Only by unearthing the true causes of discrimination can we build algorithms that correct for these.
Read the full Nature article.
___
Joshua Loftus is Assistant Professor of Information, Operations and Management Sciences