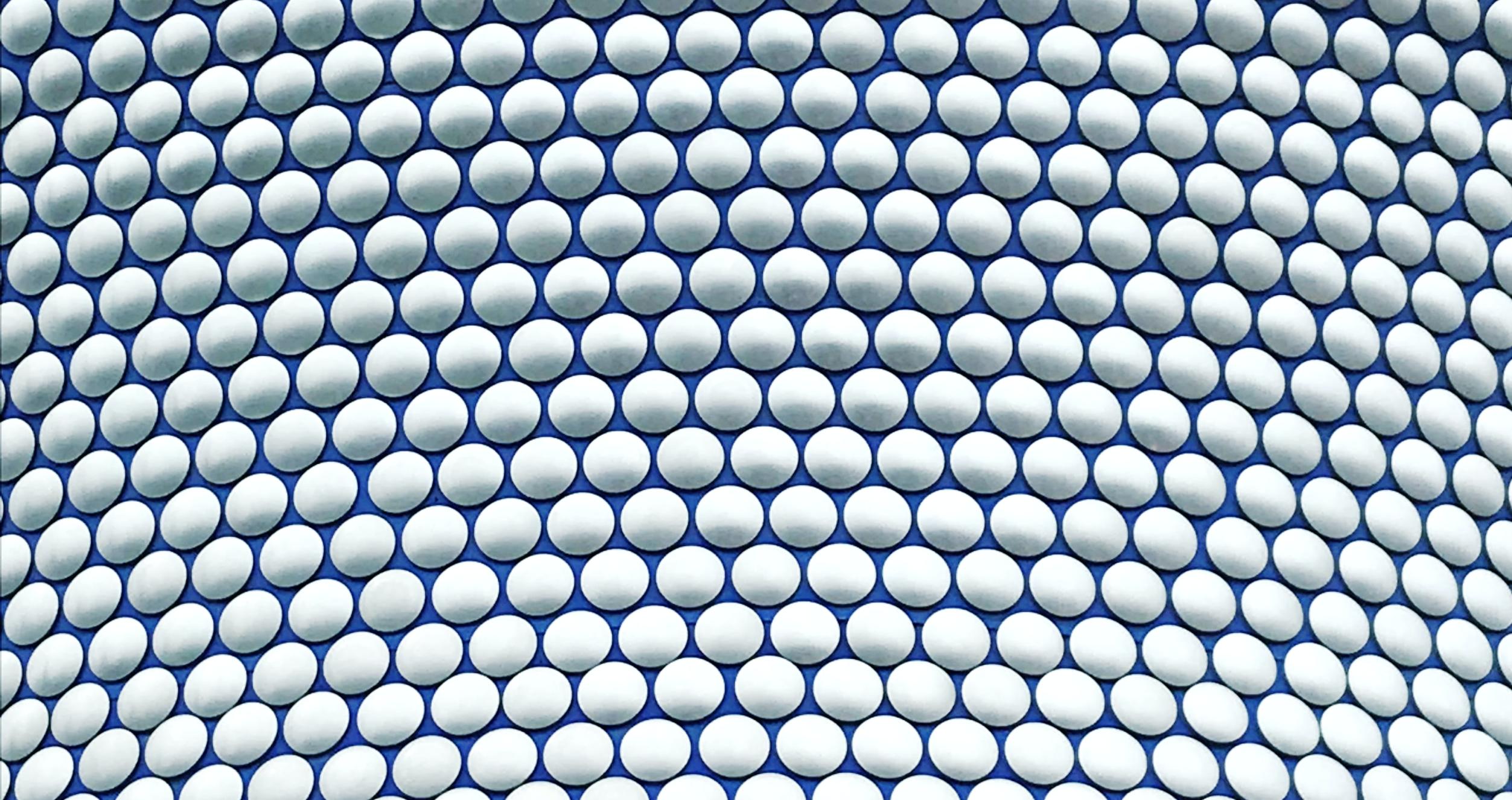
Technological advancement in the field of AI promises continuous improvements in problem-solving, perception, and reasoning that are edging closer to human capabilities. Such technologies are being increasingly adopted in contexts where typically a highly trained professional was responsible for acquiring and applying expertise applied towards making critical judgments. Recent advances in deep learning and image recognition have spurred vibrant interest and investment in developing AI research and applications for medical diagnosis based on radiological imaging. Amidst exploding expectations and claims that AI tools’ performance is superior to humans, my research seeks to understand how AI tools will be evaluated, implemented, and adopted in healthcare organizations and by medical professionals. This research is based on a year-long ethnographic study across multiple sections of diagnostic radiology within a major academic hospital at the cutting-edge of developing and adopting AI tools for medical diagnosis.
In one study, I investigate physicians’ diagnostic process in three specific settings in which AI tools were available to aid diagnosis: breast cancer, lung cancer, and bone age. I describe how physicians effortfully worked to reduce the intense ambiguity they faced in the diagnosis process and how the use of AI tools introduced additional ambiguity into the process. Of three settings in the study, only in one (lung cancer diagnosis), were AI results meaningfully incorporated into physicians’ final diagnoses on a regular basis; in the other two (breast cancer diagnosis and bone age diagnosis), AI results were largely dismissed. I discuss the key aspects of professionals’ judgment formation practices that became relevant when they considered whether and how to incorporate AI results into their diagnosis judgments, for which they must take full legal, professional, and moral responsibility. This study contributes to the nascent understanding of how AI technologies are being adopted and used in professionals’ work practices.
In the second study, I focus on the challenges that firm leaders face in deciphering complex technical materials when making decisions about which new technologies to adopt. This paper examines how organizational leaders go about evaluating the explosion of AI tools that are promising to improve accuracy and speed of decision making in organizations. I find that organizational leaders tend to focus on specific types of performance claims throughout their evaluation which emphasize certain AI characteristics (e.g., anticipated time savings or quality improvements) and ignore others (e.g., what ground truth measures were used to train and validate the model). This evaluation process has implications for which AI tools are ultimately adopted and the organizational value they create. As a result of this research, we recognize the need for technology developers to provide more transparency in how AI models were validated and for professional and organizational communities to develop more comprehensive methods and standards for evaluating AI tools.