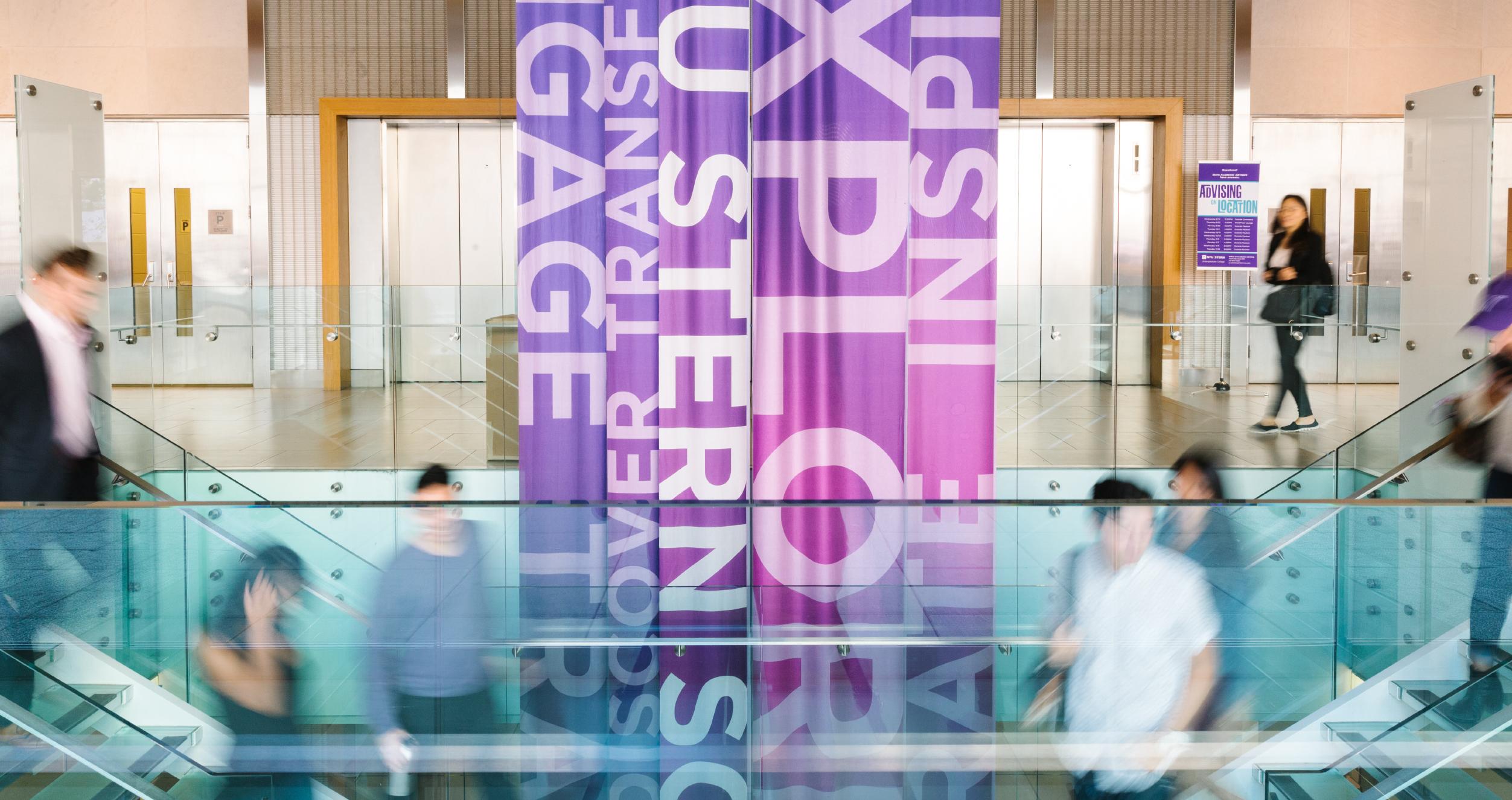
Research
Our professors publish in top Management journals in Organizational Behavior, Organizational Theory, Strategy, and Entrepreneurship. They are routinely referenced in news articles, and are recognized for their research and teaching.
A Statement About Our Research:
Our department has a longstanding, widely known reputation for innovative, rigorous and impactful scholarly research. Indeed, we are one of the top-ranked departments in the world in terms of research productivity and quality, and members of our department frequently publish their research in the most prestigious and selective academic journals. Some notable recent research publications by members of our department can be found below.